An artificial intelligence approach to remotely assess pale lichen biomass
Erlandsson, Rasmus Ingel; Bjerke, Jarle W.; Finne, Eirik Aasmo; Myneni, Ranga B.; Piao, Shilong; Wang, Xuhui; Virtanen, Tarmo; Räsänen, Aleksi; Kumpula, Timo; Kolari, Tiina H.M.; Tahvanainen, Teemu; Tømmervik, Hans
Peer reviewed, Journal article
Published version
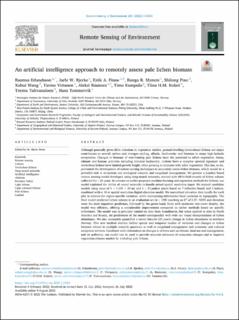
Åpne
Permanent lenke
https://hdl.handle.net/11250/3011572Utgivelsesdato
2022Metadata
Vis full innførselSamlinger
- Publikasjoner fra CRIStin - NINA [2364]
- Scientific publications [1392]
Originalversjon
10.1016/j.rse.2022.113201Sammendrag
Although generally given little attention in vegetation studies, ground-dwelling (terricolous) lichens are major contributors to overall carbon and nitrogen cycling, albedo, biodiversity and biomass in many high-latitude ecosystems. Changes in biomass of mat-forming pale lichens have the potential to affect vegetation, fauna, climate and human activities including reindeer husbandry. Lichens have a complex spectral signature and terricolous lichens have limited growth height, often growing in mixtures with taller vegetation. This has, so far, prevented the development of remote sensing techniques to accurately assess lichen biomass, which would be a powerful tool in ecosystem and ecological research and rangeland management. We present a Landsat based remote sensing model developed using deep neural networks, trained with 8914 field records of lichen volume collected for >20 years. In contrast to earlier proposed machine learning and regression methods for lichens, our model exploited the ability of neural networks to handle mixed spatial resolution input. We trained candidate models using input of 1 × 1 (30 × 30 m) and 3 × 3 Landsat pixels based on 7 reflective bands and 3 indices, combined with a 10 m spatial resolution digital elevation model. We normalised elevation data locally for each plot to remove the region-specific variation, while maintaining informative local variation in topography. The final model predicted lichen volume in an evaluation set (n = 159) reaching an R2 of 0.57. NDVI and elevation were the most important predictors, followed by the green band. Even with moderate tree cover density, the model was efficient, offering a considerable improvement compared to earlier methods based on specific reflectance. The model was in principle trained on data from Scandinavia, but when applied to sites in North America and Russia, the predictions of the model corresponded well with our visual interpretations of lichen abundance. We also accurately quantified a recent historic (35 years) change in lichen abundance in northern Norway. This new method enables further spatial and temporal studies of variation and changes in lichen biomass related to multiple research questions as well as rangeland management and economic and cultural ecosystem services. Combined with information on changes in drivers such as climate, land use and management, and air pollution, our model can be used to provide accurate estimates of ecosystem changes and to improve vegetation-climate models by including pale lichens
Tidsskrift
Remote Sensing of EnvironmentOpphavsrett
© 2022 The AuthorsBeslektede innførsler
Viser innførsler beslektet ved tittel, forfatter og emneord.
-
Feasibility of active handheld NDVI sensors for monitoring of lichen ground cover
Erlandsson, Rasmus; Arneberg, Marit Klemetsen; Tømmervik, Hans; Finne, Eirik Aasmo; Nilsen, Lennart; Bjerke, Jarle W. (Peer reviewed; Journal article, 2023)Vegetation indices are corner stones in vegetation monitoring. However, previous field studies on lichens and NDVI have been based on passive sensors. Active handheld sensors, with their own light sources, enables high- ... -
Kartlegging og overvåking av rødlistearter. Arealer for Rødlistearter - Kartlegging og Overvåking (ARKO)
Sverdrup-Thygeson, Anne; Bakkestuen, Vegar; Bjureke, Kristina; Bratli, H; Framstad, Erik; Skarpaas, O; Stabbetorp, O; Wollan, Anders; Ødegaard, Frode (NINA Rapport;528, Research report, 2009)Sverdrup-Thygeson, A., Bakkestuen, V., Bjureke, K., Blom, H., Brandrud, T.E., Bratli, H., Endrestøl, A., Framstad, E., Jordal, J.B., Skarpaas, O., Stabbetorp, O.E., Wollan, A.K. & Ødegaard, F. 2009. Kartlegging og ... -
Gjenanalyse av intensive overvåkingsfelter for markvegetasjon i Endalen, Svalbard 2014. Endringer i vegetasjon fra 2009 til 2014 og vurdering av overvåkingsmetodikk
Bakkestuen, Vegar; Aarrestad, Per Arild; Stabbetorp, Odd Egil (NINA Rapport;1122, Research report, 2015-06-30)Bakkestuen, V., Aarrestad, P.A. & Stabbetorp O.E. 2015. Gjenanalyse av intensive overvåkingsfelter for markvegetasjon i Endalen, Svalbard 2014. Endringer i vegetasjon fra 2009 til 2014 og vurdering av overvåkingsmetodikk ...